Revolutionizing Finance: The Impact and Use Cases of AI in the Financial Sector
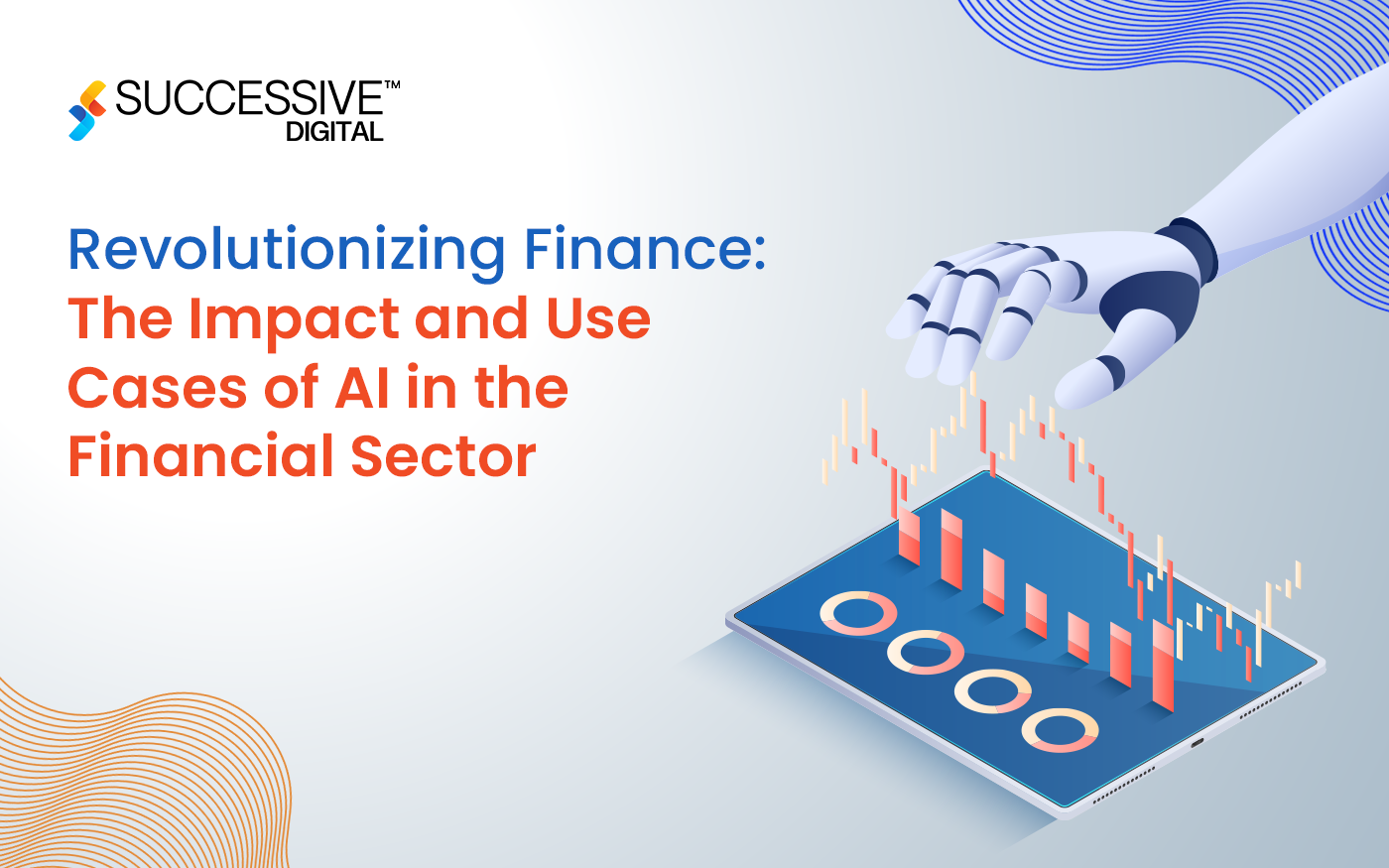
Impact of AI in the Finance Sector
The finance sector is at a pivotal moment. The industry has undergone seismic shifts in recent years, largely due to the rapid growth and adoption of artificial intelligence (AI) technologies, including large language models (LLMs). Technology disruption and consumer shifts are laying the basis for the adoption of new technology for financial business models, and the COVID-19 pandemic has accelerated these trends. Building upon this momentum, the advancement of artificial intelligence (AI) technologies within financial services offers these organizations the potential to increase revenue at lower operating cost by engaging and serving customers in radically new ways.
AI has proven invaluable in the financial services industry as it empowers organizations to optimize operations, elevate customer service and pinpoint potential risks.
This article collected the key milestones on a path we believe can lead the financial sector to deeper customer relationships, expanded market share, and stronger financial performance by leveraging the power of AI.
AI and Financial Institutions
Businesses, at large, are integrating artificial intelligence (AI) into their everyday operations. In finance, it supports lending decisions, fraud detection, and customer personalization. It also drives business process automation that helps financial institutions deliver a better customer experience. Over several decades, financial institutions have continually adapted the latest technology innovations to redefine how they interact with their customers. ATMs were introduced in the 1960s, and electronic, card-based payments were introduced in the ’70s. The 2000s saw the broad adoption of 24/7 online banking, followed by the adoption of mobile-based fintech app development capabilities through “banking on the go” in the 2010s. Fast forward to 2023, these technologies are yielding results higher than expected for the market.
The Credit Cards and Ultra Precise Marketing
The chances are high that the credit card you are using was applied after seeing an advertisement on a particular application or a credit card executive sign you up for a credit card at a mall. Or you may have simply gone through the bank or credit union you already do your transactions with. But all these cards and their benefits are generalized and do not often meet with the user’s spending habits.
Now, AI can help credit card issuers get the right card into the right hands by drilling into the data of user’s credit reports to ensure they get highly personalized credit offers.
AI-driven models are based not just on user’s credit history and shopping habits but also on what the issuer is looking to offer to a cardholder.
For example, the issuer may want to identify people who have had no late payments over the past five years or have kept their credit utilization ratio below a certain percentage.
As for you, AI can do things such as pinpoint how you prefer to access your account’s rewards and whether you are using the tools on the card’s app. With that information, the issuer can present you with an account that fits both their qualification standards and your usage history.
AI can manipulate the pricing of the product and the way we use our credit cards. The AI models overlay our transaction data and other involved information, including the type of product purchased, payment method, and customer demographics. To make it interesting, these models add third-party data on inputs such as weather and location at the point of purchase using the already-fetched data.
The result – a projection of the optimal pricing for an age group of 18 to 25-year-old customer who is using credit cards to buy gifts for festivals when the weather is poor.
Artificial Intelligence has penetrated day-to-day lives and becomes an integral part of the most demanding and fast-paced industries.
Forward-thinking executive managers and business owners actively explore new AI use cases in finance and other areas to get a competitive edge on the market. When the financial sector blends its offerings with immersive technology, here are the ways the output becomes much more fruitful.
Also read – FinTech App Development Guide
Boosts Revenue
AI technologies can help boost financial institution revenues through increased personalization of services and fostering innovation in customer experience. Here are some specific ways in which AI can contribute to revenue growth in the industry.
Personalized Customer Experience:
When AI-powered chatbots engage with customers in real-time, providing personalized assistance and resolving queries promptly, the customer experience is enhanced, increasing customer satisfaction & loyalty, boost up customer retention and repeat business.
Product and Service Recommendations:
AI algorithms review customer data, transaction histories, and preferences to offer personalized product and service recommendations tailored to their interest; this results in higher conversion rates and higher revenue.
Credit Risk Assessment:
With the use of AI, financial institutions assess credit risks more accurately and efficiently. This enables them to make better lending decisions, increasing the likelihood of approving loans to creditworthy borrowers and reducing defaults. (View section: AI and Credit Risk Assessment)
Automated Customer Onboarding:
AI-powered processes can automate customer onboarding, making it quicker and more efficient. This leads to a faster customer acquisition process and a reduction in customer abandonment rates, ultimately boosting revenue.
Optimized Pricing and Product Bundling:
By offering the right products to the right customers at the right price, banks can increase sales and revenue. AI can analyze customer behavior and market trends to optimize pricing strategies and product bundling.
Lowers Cost
Automating Repetitive Tasks:
These models can automate routine and repetitive tasks like data entry, transaction processing, and document verification. By reducing manual labor, many financial institutions can save time, minimize errors, and cut operational costs.
Risk Management:
AI assists financial institutions in accessing credit risk, market risk, and operational risk more accurately and quickly. By having better risk assessment models, financial institutions make more informed decisions, leading to reduced losses and improved capital allocation.
Customer Service and Chatbots:
AI-driven chatbots and virtual assistants can handle customer queries and complaints round-the-clock, reducing the need for human customer service representatives. This leads to cost savings in staffing and training.
Optimizing Investment Portfolios:
Algorithms are a big part of investment and stock trading; AI-backed models enable firms to optimize investment portfolios, lowering costs related to asset management and potentially increasing returns for customers. (View section: AI in algorithmic trading)
Uncovers New Opportunities
AI is helping financial institutions unlock new opportunities based on an improved ability to process and generate insights from vast troves of data. They are now more focused on using technology to deepen customer relationships.
For example, designing intelligent value propositions that solve unmet needs, harnessing big data to deliver hyper-personalized services and enhanced cross-selling, and improving financial performance.
In addition to having an AI-first mindset, financial institutions are also ensuring that these AI capabilities work to their advantage and deliver actual business value.
AI in Fraud Detection
As competition for credit relationships becomes concentrated in digital channels, the automated processing of loan applications and the use of AI/ML models to expedite credit approval and disbursement of funds not only positions the financial institutions to acquire new customers and increase market share but also opens new opportunities for fraud detection.
The costliest instances of fraud typically fall into one of five categories: identity theft, employee fraud, third-party or partner fraud (e.g., fraud by sales agents), customer fraud, and payment fraud (including money laundering and sanctions violations).
This results in an overall cost increase. Every $1 lost to fraud now costs U.S. financial services firms $4.23 and this cost continues to increase. Not only do the frauds impact financial institutions, but as more and more young adults are falling prey to the increasing scams, these frauds cost consumers $8.8 billion per year.
How do AI Fraud Detection Models Work?
AI is enhancing the safety of financial services by easy fraud detection, using its capabilities in data analysis, pattern recognition, and real-time monitoring.
Here is How AI-driven Fraud Detection and Prevention Models Work:
AI models can detect and flag anomalies in the user’s spending patterns in real-time. These models start by gathering, processing, and categorizing historical data. This includes a bifurcation of ‘good data,’ which is the labeled information about legitimate transactions, and ‘bad data,’ information about fraudulent transactions.
After this, data engineers feed the machine with varied examples of banking fraud patterns to make the algorithm agile, versatile, business-specific, and real-time. As a result, whenever a user makes a new transaction, the data feeds back into the system, and self-learning and adaptive analytics enable the machine to incorporate the new data while adjusting to the changing fraud environment, allowing it to recognize new forms of fraud.
Ways AI can Help Detect a Fraud
- Real-time Monitoring: Enabling immediate detection of suspicious behavior and potential fraud attempts. This real-time monitoring allows financial institutions to respond quickly and prevent losses.
- Behavioral Analysis: To understand the typical behavior of individual customers over time by considering factors like spending habits, transaction frequency, location, and device usage. When deviations occur, AI can raise alerts for further investigation.
- Transaction Link Analysis: To perform network analysis to identify connections between different accounts or customers engaging in fraudulent activities, helping financial institutions uncover organized fraud rings.
- Machine Learning Models: To build and train machine learning models on historical fraud data, enabling the system to continuously improve its fraud detection accuracy as it encounters new patterns.
- Big Data Analysis: To analyze vast amounts of data from diverse sources, including transaction records, customer profiles, social media data, and more, to identify suspicious patterns and connections.
- Biometric Authentication: Adding an extra layer of security to ensure the legitimacy of customer identities and transactions.
- Predictive Analytics: To predict potential fraud attempts based on historical data and trends, helping financial institutions take proactive measures to prevent fraud before it occurs.
AI and Credit Risk Assessment
Artificial intelligence develops sophisticated risk models by analyzing vast amounts of historical data, market trends, and other relevant factors and can potentially help financial institutions with credit assessment. AI can build and train machine learning models on historical credit data to predict the likelihood of default based on various risk factors. Machine learning algorithms can identify patterns, correlations, and hidden risks that traditional risk assessment methods might miss. This gives financial institutions a leverage to make more accurate predictions for credit payers and assess the potential dangers across various asset classes.
How Does That Work?
AI-driven models evaluate the creditworthiness of borrowers to determine the likelihood of defaulting on loan obligations. Through the credit risk assessment process, AI-driven credit softwares analyze the creditworthiness based on a certain parameters (such as cutoff points) and the model outputs by automatically approve or deny the applicants.
These machine-learning models use historical fraudulent and non-fraudulent data related to transaction amounts, merchant information, geographical location, and the date/time of the transactions. The models will be fed known fraudulent transactions as well as legitimate ones.
Data engineers will then process and pre-clean the data to ensure that the data quality is high caliber.
Ways AI can Assess the Credit Risk
- Real-time Monitoring: Continuously monitoring borrower behavior and financial indicators, providing real-time updates on credit risk changes and helping lenders take timely actions.
- Automated Decision-Making: Reducing human bias and processing times while ensuring consistency and adherence to predefined risk criteria.
- Behavioral Analysis: Analysis of customer behavior patterns to identify any shifts or red flags that might indicate potential credit risk, even for existing borrowers.
- Portfolio Analysis: Overall evaluation risk of a lender’s credit portfolio by analyzing the distribution of risk levels and identifying concentration risks.
- Predictive Analytics: Predicting the probability of default for specific loan applicants, helping lenders make more informed decisions on loan approvals and pricing.
- Early Warning Systems: Creation of early warning systems that flag deteriorating credit conditions for borrowers, enabling lenders to take proactive measures and mitigate potential losses.
Personalized Wealth Management with AI
The adoption of AI-powered technologies in the finance sector empowers individuals to take control of their finances, make informed decisions, and build a secure future tailored to their unique needs. As a result, AI-powered personalized financial planning is at the forefront.
As mentioned before, AI analyzes the already fed data, understand patterns, and generate insights beyond human capabilities. This enables wealth managers to create personalized investment strategies, identify potential risks, and optimize portfolio performance precisely.
Customized Financial Plans with AI
AI-powered financial planning offers many benefits, but the biggest of all is its ability to understand users’ unique aspirations and risk tolerance. Through interactive platforms and intuitive interfaces, AI-driven tools can gather essential information about your financial goals, time horizon, income, and expenses. This data fuels the AI’s algorithm, which then crafts a personalized financial plan tailored to your specific needs.
For example:
Imagine a user is planning on buying a property in the next five years. The AI-powered models will assess the current financial status and inflation rates over the next five years and analyze the real estate market to create a step-by-step roadmap toward owning a property in the next five years. This level of personalized guidance was once the privilege of the ultra-wealthy, but now, AI brings it within reach for everyday individuals.
Benefits of AI-powered Wealth Management
Investment Strategies:
Easy analysis of user’s financial data, goals, and risk profile to create customized investment strategies that align with their objectives and preferences.
Robo-Advisors:
Automated investment advice, portfolio management, and rebalancing based on real-time market data and the client’s financial objectives.
Risk Assessment:
Assessing users’ risk tolerance and dynamically adjusting investment portfolios to match their risk appetite, helping to achieve a balance between risk and potential returns.
Behavioral Finance Insights:
Analysis of user’s behavior and emotional responses to market fluctuations, enabling wealth managers to provide behavioral finance insights and prevent irrational investment decisions.
Continuous Monitoring:
Continuous monitoring of financial markets, economic indicators, and individual portfolio performance to make real-time adjustments to optimize returns and manage risks.
Tax Optimization:
Helps with tax-efficient investing, optimizing investment strategies to minimize tax liabilities for clients.
Forecasting and Management of Bad Debt with AI
Debts turning bad is the soaring problem plaguing any country’s economy at large. It reduces the financial institution’s profitability and limits its ability to issue new credit. They also risk hampering long-term economic growth, leading to greater uncertainty in the system and, in turn, elevated financial stability risks.
According to the US tax authorities, bad debt accounts for 0.5% of US firms’ revenues.
In 2018, over US$100 billion was missing money, reducing profit margins by as much as 5%2.
AI can significantly enhance the accuracy and efficiency of bad debt forecasting and management by leveraging its data analysis and predictive capabilities.
How Does It Work?
Based on the analytics by artificial intelligence, financial institutions gain the ability to predict which customers will pay, be late in paying, or will not pay at all.
By harnessing the power of AI and machine learning, institutions can spot the early warning signs of soon-to-be-defaulting accounts. AI-backed models let the host of data sources spot signals of credit risk before they were self-evident. Credit risk signals would indicate that a particular customer stood a strong chance of defaulting on payment.
An ML model can draw insights from vast pools of data that lay beyond the capabilities of human resourcefulness and analyze millions of collected data across many sources, including customer data, expenditure patterns, historical invoice payments, store data, etc. Having access to these early warning signs, a financial organization can then take steps to intervene before a likely problem becomes a crisis.
For Example:
A financial service-offering institution was suffering from higher-than-average bad debts. The team rushed to the manual analysis to find patterns of the non-payments. The firm made the analysis across home type, down payment, and credit standings and found no discrepancies. However, they later built a predictive model that included the pre-fetched data from CRM and had a correlation with the approved loans by the firms. The model built on machine learning analyzed the ongoing relationships between certain compensation structures and bad debt, providing a long-term forecast of defaults under different scenarios.
AI in Generating Financial Reports
As we talk about financial reporting, it’s indeed one of the most crucial functions for any business, but it can also be a time-consuming and error-prone process. Manual data entry, complex calculations, multiple sources and formats, and changing regulations can create challenges for accuracy, efficiency, and compliance.
AI-powered systems can generate financial reports automatically based on predefined templates and formats. These systems can extract relevant data, perform calculations, apply accounting rules, and produce comprehensive reports without human intervention.
Automated report generation allows for increased agility and flexibility, as reports can be generated on-demand or scheduled to run at specific intervals, providing real-time insights for decision-making. This streamlined process enhances.
- Overall efficiency
- Improves data integrity
- Empowers organizations to make informed financial decisions promptly.
Here are some ways that AI can transform financial reporting and add value to your business.
Automated Data Collection:
One of the most tedious and labor-intensive tasks in financial reporting is collecting and validating data from various sources, such as ERP systems, spreadsheets, invoices, bank statements, and tax forms. AI can automate this process, classify, and verify data from structured and unstructured documents.
Data Validation and Accuracy:
AI models can help financial institutions with data validation. With the use of various algorithms, AI can validate financial data for accuracy and completeness, ensuring the reliability of financial reports and reducing the risk of errors.
Enhanced Data Quality and Compliance:
AI can also use semantic analysis and knowledge graphs to map and align data with different taxonomies, ontologies, and frameworks and update data according to the changing rules and requirements.
Also read – Digital Wallet App Development Guide
AI in Algorithmic Trading
Algorithmic trading is the practice of purchasing or trading security according to some prescribed set of rules tested on past or historical data. Algorithmic trading has transformed the way trading is done. With the use of AI, we can anticipate algo trading to move into a more pragmatic machine learning form that can manage real-time deciphering of large volumes of data from many different sources.
AI has significantly impacted algorithmic trading, revolutionizing the way financial markets operate. Algorithmic trading, also known as algo-trading, is the use of computer algorithms to automate the process of executing trading orders in financial markets.
In the form of online trading apps, AI-backed models help unlock profitable opportunities for users while minimizing investment risks simultaneously. Financial firms can get valuable insights into complex trading opportunities that support real-time buy and sell decisions by analyzing stock prices continuously and processing massive amounts of unstructured data.
One of the most important advantages of AI for algorithmic trading is its ability to process massive amounts of data in real-time. This is especially important in financial markets, where prices and trends can shift quickly and unexpectedly. Traders can quickly analyze vast amounts of market data and make informed trading decisions based on the most up-to-date information by using AI-powered algorithms.
Here are the Ways AI can Transform the Stock Trading
Pattern Recognition:
AI-powered models can analyze historical market data to identify patterns, trends, and correlations that may indicate potential trading opportunities. These patterns might be difficult for human traders to identify due to the sheer volume and complexity of the data.
Predictive Analytics:
Through predictive analytics, these models can make predictions about future market movements based on historical data and real-time market conditions. This helps traders make more informed decisions about when to buy or sell assets.
Sentiment Analysis:
AI-driven sentiment analysis can process and interpret news articles, social media posts, and other unstructured data to gauge market sentiment and its potential impact on asset prices.
High-Frequency Trading:
AI algorithms enable high-frequency trading by executing a large number of orders at extremely high speeds, leveraging ultra-low latency connections to exchanges.
Quantitative Trading Strategies:
Based on statistical analysis and historical data, AI models can develop robust trading strategies, enabling traders to execute high-frequency, systematic, and rule-based trading strategies.
Final Words
The finance industry has already uncovered valuable ways to live with and work with AI, but it will take time, patience, refinement, and some creativity to leverage it to its maximum potential in the services offered by this sector. By embracing immersive technologies, while taking the necessary precautions, financial institutions can stay ahead in a competitive landscape, ensuring they continue to cater to their customer’s evolving needs. As we talk about AI, Successive Digital prioritizes business success and offers technological feasibility. With more than 5000 success stories in “innovation with AI,” Successive Digital continues to provide the best in market mobile app development services, including MVP development for beginners.
If you want Successive Digital to help you with AI adoption in the finance industry, feel free to contact us.
Search
Recent Posts
-
6 Proven Tips for Effective DevOps Collaboration and Communication
-
Fintech App Development Trends
-
Why Should You Choose Acquia CMS For Headless Website Development?
-
How Fintech Applications Are Transforming Banking And Financial Services Industry
-
5 Essential Fintech App Development Features to Improve Your Customer Experience